Between handshakes, AI panels, and a feverish haze, I dropped the ball. To those who caught it, a bit thank you for being patient.
By the afternoon of March 13th, I was barely holding it together.
Still, I dragged myself back to ExCeL London. A promise was a promise, especially when I agreed to chair sessions with industry leaders flying in from across Europe.
For my American readers wondering what the fuss is about: Tech Show London is to Europe what RSA or AWS re:Invent is to the US tech scene, just smaller (as most other things). This year, about 25,000 attendees attended London, one of the must-attend annual tech events on the continent.
TL;DR
- As GPUs replace CPUs. While everyone obsesses over model parameters, The hot topics in the data center section are cooling and power constraints.
- Packed conference sessions discussed autonomous agents. The agentic AI drama continues and remains as is: talk.
- My talk challenged the AI certainty bubble through nine real-world cases that transformed marketing headlines into uncomfortable questions.
- Panels showcased ambitious AI roadmaps, while private discussions acknowledged pilots that never reached production. A clear implementation gap.
- Can’t build an AI castle on a data swampland. From my fireside chat with Merve Alanyali to data scientists across multiple sessions, the emphasis was on fundamentals over algorithmic excitement.
- The training paradox is on full display. Vendors eagerly sell courses on technologies so nascent they lack established patterns. But hey, what’s more important than slipping in the term AI for your enterprise training package?
Physics Doesn’t Care About Your AI Sales Pitch.
If you ask me,
What’s actually exciting at the show?
My first reply would be:
Data centers are getting hotter.
While many in my network (or general media) obsess over model parameters and agentic AI. A good reminder is that one of AI’s fundamental constraints is physical.
The shift from CPUs to GPUs for AI workloads creates problems that no amount of venture funding can solve:
- Power requirements spiking beyond existing grid capacities
- Thermal management hitting thresholds, physics doesn’t negotiate
- Infrastructure costs are piling up.
As GPU fundamentally needs more power and runs hotter.
In the data center section, many innovations focused on “improved cooling, improved power density.” It’s not exactly the sexy AI narrative tech journalists typically chase, but a model w/o a physical foundation will find it hard to expand.
Mass Rebranding Exercise. New Lable: AI
Walking the software aisles felt like watching a massive rebranding operation in real-time. It was like an expensive annual costume party where everyone arrived wearing the same mask.
The cybersecurity section was particularly illuminating. Two years ago, these exact systems proudly wore the “machine learning” label. Last week, they were all “AI-powered cybersecurity” solutions. Same detection algorithms. Same anomaly identification logic. Fresh buzzword.
How do you find out that certain network traffic is or isn’t as expected, or dangerous, or different from normal?
One vendor pitched. That question hasn’t changed in a decade; only the marketing terminology used to answer it.
The Microsoft ecosystem displayed particular shamelessness. Partners “incentivized to sell you more Microsoft stuff whether you need it or not” simply slapped “AI-enhanced” labels on the same licensing packages they’ve pushed for years. Again, it is a standard Microsoft playbook with fresh branding.
That said, I’m excited about the potential breakthrough with the production environment digital twin.
A demonstration caught my attention. It used “broadly AI, not just gen AI” to create replicated environments. This is something interesting that could address a persistent engineering challenge: recreating environments that mirror production, including traffic patterns and load characteristics.
Still, there is a lack of technical detail about exactly how they achieved this.
The Agentic AI Reality Check
I attended a panel, and part of the discussion was about agentic AI.
One panelist was full of drive and promoted their agent-based approaches, but the conversation eventually forced them to this reluctant admission: we have no production use cases.
One candid CTO finally conceded:
There is little real-world commercial Agentic AI examples… no proper playbook.
This implementation dilemma transcends mere technical challenges.
Here’s a short takeaway, but I plan to turn this into something proper in the next few months: There is a fundamental economic contradiction at the heart of agentic AI. The more autonomy you grant, the less reliable your system becomes.
Either you build an agent with too many errors for production use, or you add so many human checkpoints that it becomes more expensive than just doing the work manually.
Challenges I see:
- Imagine this… if each autonomous step introduces its error probability (hallucinations). A 1% hallucination rate compounds to a 40% failure rate after 50 steps, what’s the realistic scope for autonomous agents in critical systems?
- How do you debug what you cannot see? When decisions emerge from opaque processes, tracing failure modes becomes an exercise in expensive guesswork rather than engineering.
- When each decision node becomes a potential exploitation target. More agents equal more vulnerability — a reality hardly discussed, not to mention being addressed.
- When multiple AI systems interact across organizational boundaries, who should be accountable for the final error?
No wonder Agentic AI deployment remains “six months away.”
Nine Patterns After Reviewed Major AI Failures
https://cdn.embedly.com/widgets/media.html?src=https%3A%2F%2Fwww.youtube.com%2Fembed%2Fkh4iZjboDf4%3Ffeature%3Doembed&display_name=YouTube&url=https%3A%2F%2Fwww.youtube.com%2Fwatch%3Fv%3Dkh4iZjboDf4&image=https%3A%2F%2Fi.ytimg.com%2Fvi%2Fkh4iZjboDf4%2Fhqdefault.jpg&type=text%2Fhtml&schema=youtube
I covered nine anecdotes across three themes in my research:
- Strategic disconnects,
- Technology limitations,
- … and human elements that most AI roadmaps ignore.
Each story is punctuated with questions designed not to test knowledge but to challenge certainty.
The research about AI deception and critical thinking erosion sparked most hallway conversations after my talk.
Most valuable were the questions I asked in the end:
- Is your AI product solving genuine user problems?
- How much do you know about your model’s limitations and the risks you are exposed to?
- How do you motivate yourself and your team to think critically still?
I strongly recommend watching the talk ☝️ (will be uploaded soon)
My friend documented how many people attended and showed genuine interest in honest AI conversations rather than hype cycles.
The Mature Voices in AI 2025. Some Positive Developments.
The 2nd day of the event kick-started with my morning fireside chat with Merve Alanyali. It struck me hard; it is precisely this kind of work hidden beneath the marketing line and the hype we need to see.
As we sat beneath the harsh conference lighting, mark her words
Getting the plumbing right is the key to success for AI transformation.
or that
We’ve heard too much about AI culture. However, to me, it’s not about lecturing the teams but bringing them along through exercises, discussions, and other well-designed activities. It’s a lot of hard work, but it’s also so much more effective.
It was one of the best fireside chats I moderated, and it was very insightful and inspiring.
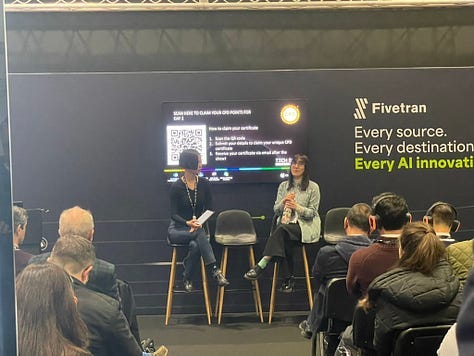
Harnessing AI for Positive Impact and Transformative Change with Merve Alanyali
The topics covered resonated with the overarching theme across the multiple sessions from people who do the work:
- Data scientists across various panels emphasized that clean, governed data creates more business value in the long run.
- Instead of rushing to an AI solution, most companies should focus on looking for the data they possess with the highest density of information value. (I should expand on this in a future post)
- The most sophisticated discussions addressed approaches spanning organizational boundaries. It is less about fancy AI facades and more about cultural readiness, skill development pathways, and governance models.
- Thoughtful practitioners now focus on deliberate engagement with regulations. Regulation should be used as a framework for responsible innovation rather than seen as a limitation.
- Cultural infrastructure: The most impactful presentations focused on transforming data literacy from specialized knowledge between teams.
These weren’t the conversations that generated dramatic headlines.
They’re too practical, too honest about limitations, and too focused on sustainable value rather than disruption narratives.
Maybe, just maybe, something we actually need in the AI industry.
My crystal ball tells me that the most successful companies, in the long run, aren’t those asking, “Which model should we implement?” But
How do we restructure our data foundation and what’s the value of our data, so we can keep the advantages whatever models emerge?
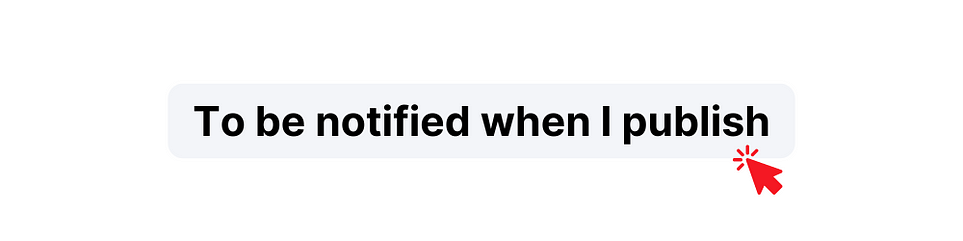
Cold Medicine and Clarity. Final Thoughts
Nothing some LEMSIP can’t fix… though by the 2nd day afternoon, it was definitely wearing off.
The most valuable insights emerged between sessions when presenters dropped their presentation personas:
- What’s running in production versus perpetual pilot?
- How are you measuring success beyond technical metrics?
- What AI capabilities deliver value today, not someday?
However, I still see a chasm between marketing lines and technical realities. The tech industry operates on cognitive dissonance between big tech CEOs and AI experts vs. the rest.
I was also really happy to hear that some practical work and human elements are starting to flourish. This event showed signs of an industry finally maturing past its adolescent hype cycle.
And that’s progress worth celebrating, even with a fever.
Source: www.medium.com